Written by Wayne Zheng in PHYSICS on Thu 21 May 2020. Tags: tensor network,
Introduction
Tensors and their contraction
Tensor is a higher-dimensional generalization of the matrix, which has two indices such as \(M_{ij}\). For example, a four-dimensional tensor can be obtained by the tensor product of two matrices \(\left(A\otimes B\right)\_{ij}=C_{ij}=C_{k\cdot d_{l}+l, m\cdot d_{n}+n}=A_{km}\cdot B_{ln}\equiv T_{mn}^{kl}\), where \(d_{l, n}\) are the dimensions of the corresponding indices. Each index of a tensor is called a bond. Any element in a rank-\(D\) tensor \(T_{n_{0}, n_{1}, \cdots, n_{D-1}}\) can be mapped to a one-dimensional vector \(v(p)\), which is the actual physical storage in a computer's memory. Generally there are two ways to do this.
- Row-major. \(p=\sum_{k=0}^{D-1}n_{k}\cdot\left(\prod_{l=k+1}^{D-1}d_{l}\right)\). The elements along the last index are contiguous. It is implemented in
numpy
as default. - Column-major. \(p=\sum_{k=0}^{D-1}n_{k}\cdot\left(\prod_{l=0}^{k-1}d_{l}\right)\). The elements along the first index are contiguous. It is implemented in Fortran and
Eigen::Tensor<>
(C++) as default.
Two different tensors can be contracted if they share a same dimension. It is a generalization of the matrix production like \(C_{ij}=\sum_{k}A_{ik}B_{kj}\). Graphically, we use a connected link to denote the connection of two bonds. Tensors with some connected and unconnected bonds can form a tensor network (TN).
Matrix product state and matrix product operator
Firstly I should point out the so-called and matrix product operator (MPO) are actually comprised of tensors rather than matrices. This is a historical issue. A one-dimensional (1D) quantum state can be written as a matrix product state (MPS):
\(A\) has already been chosen to be left-canonical, namely \(\sum_{j}A^{j\dagger}A^{j}=1\). The basic idea of MPO is to introduce more inner bonds to decouple the operation of a many-body operator with finite range interactions into the production of local onsite tensors.
A matrix product operator (MPO) looks like
\(\gamma_{0}=1\) denotes a virtual bond.
One of the simplest physical TN is to compute the expectation value of a MPO in terms a MPS like \(\langle\psi|H|\psi\rangle\), which can be expressed as
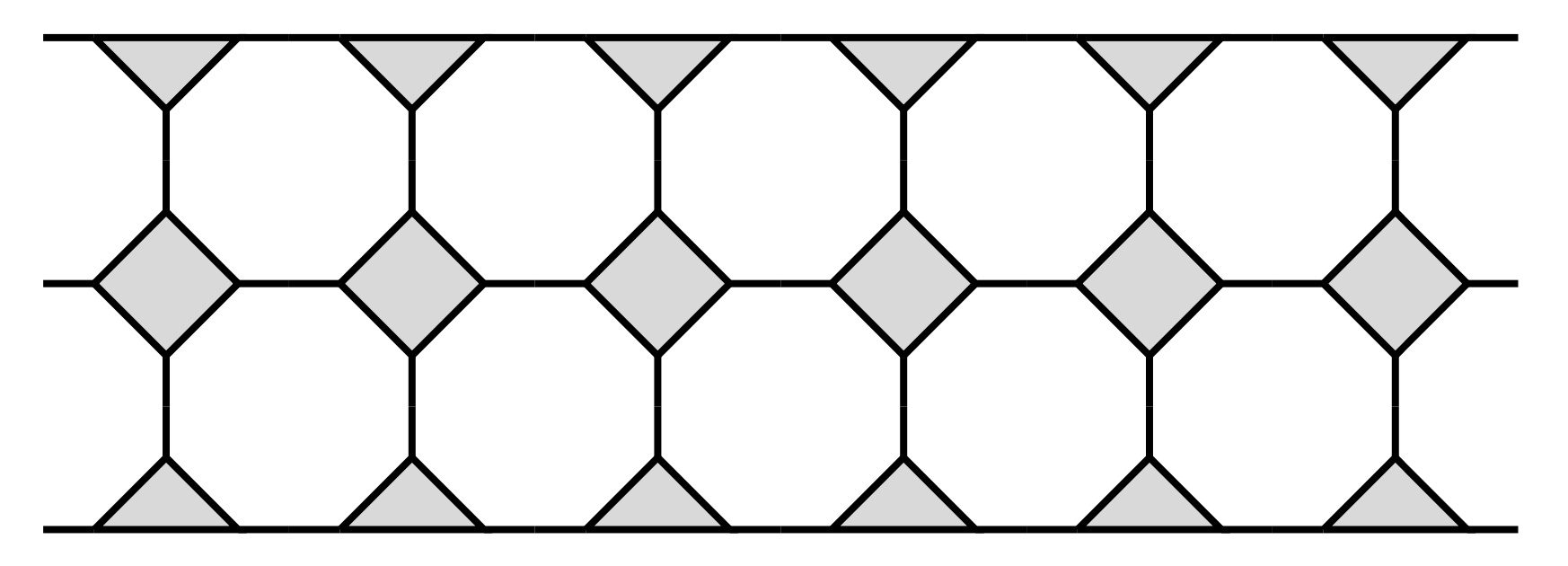
How to practically contract a TN
An example
Here we consider a TN \(\langle\psi|H|\psi\rangle\) as an example, in which \(H\) is chosen to be the XYZ-model
Written into a MPO
we have several methods to contract this TN:
-
Naive method (NM). By a naive way, we can paste all the tensors piece by piece utilizing
numpy.tensordot
. On site \(j\), we can paste the upper MPS, MPO and lower mps into a rank-6 onsite tensor. Then paste it to the environment tensor, which has three virtual bonds. As a member function of the MPO, it looks like: -
Optimized
einsum
(OE). By utilizingopt_einsum
1, we can speed up the contraction dramatically, which could find the optimal contraction order automatically. At each site \(j\), we can contract the environment, upper MPS, MPO and lower MPS at the same time. Moreover, it supports CUDA throughCuPy
. -
Optimized
einsum
as a whole graph. Instead of site by site, we can pass all the tensors and contracting information toopt_einsum
, which can handle the whole TN and find out the optimal contracting path. This method will be particularly useful to contracting a two-dimensional TN.
Numerical results
Platform: Intel(R) Xeon(R) CPU E5-2640, NVIDIA Tesla P100, CUDA 9.2.88. The XYZ-Hamiltonian is chosen as \(J_{x}=J_{y}=1.0, J_{z}=h=0.5\). The MPS is generated randomly.
We conclude that:
-
By utilizing
opt_einsum
in stead of the naivetensordot
, the contraction can be dramatically speeded up \(10^{3}\) times as much as possible especially with large bond dimension \(\chi\). With relatively small \(\chi\), CUDA-OE is even slower than OE, while not too much. -
CUDA
can further speed up about as many as \(10^{2}\) times. It reaches the threshold at about \(\chi\simeq 250\), below which we think that the cores in GPU are not fully implemented. Overall, if we useopt_einsum
combined withCUDA
, we can speed up the tensor contraction as many as \(10^{5}\) times in comparison with the naivetensordot
.
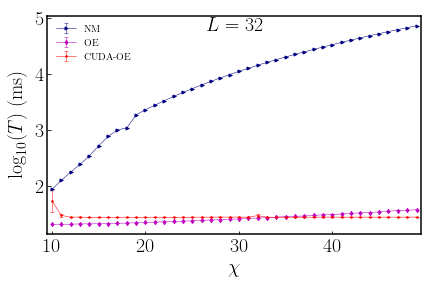
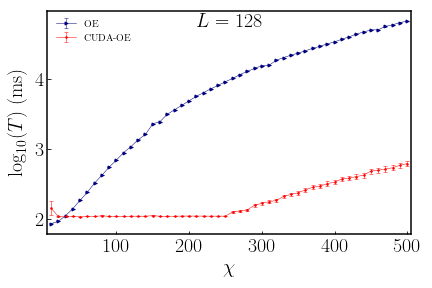
The original notebook and code can be found here.